AI Powered Underwriting: Tackling the Data Challenge.
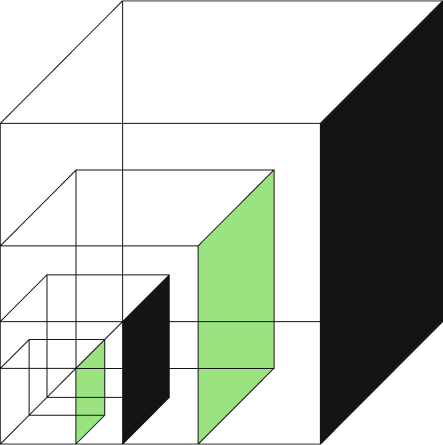
Data Flaws Detrimental to the Underwriting Process.
The ability to rapidly react to both internal and external factors, simplifying the bordereaux process and achieving data perfection are some of the key objectives for our underwriting clients. Reaching a level of holistic business understanding that go beyond analytics being the ultimate goal. In many cases they have identified AI tools that they want to use to help them achieve this goal but their data is not AI ready, meaning they cannot trust AI outcomes in the way they need to.
The Problems:
- Bordereaux data being received in multiple formats.
- Data sources disjointed, requiring a lot of moving data between systems for comprehensive overviews.
- Consolidation and compilation of data was time consuming and labour intensive
- Over-reliance on manual clean up leading to data flaws.
- Data flaws progressing through data pipelines and impacting end analytics.
- Report building and analytics requiring deep tech expertise due to volumes.
- Decision making based on misinformation.
- Over reliance on personal experience and ‘instinct’ for assessments.
- Inability to embrace new technologies due to complexity of historic data structures.
- Trying to balance between innovation and regulatory and compliance restrictions.
- Not having a quick and simple way to get a holistic business understanding.
By using the Lean Data for AI framework, our underwriting clients document both the business context and key problems they want solved. Once this initial business context and problem have been characterised these details can be shared with a generative AI tool to define the data needed for the business problem.
For underwriting teams, this might be key data sets from right across their business, cover holders or even public data like or market changes or valuations. AI has helped speed up this documentation stage but this is where traditional data management either spirals into long, tech heavy projects or simply fail completely. Verodat supports a different path.
AI Readiness by Design
Having identified clear objectives and understanding the underlying data needed to meet these objectives, our underwriting users then publish the data model and the requirements to the Verodat platform.
A key stage in the Lean Data Management for AI framework, they focused solely on the data they needed rather than the data they had. This allowed them to quickly pinpoint both gaps to be filled and opportunities for added business value.
As a data supply chain platform, Verodat facilitated the sharing of these requirements with the information owners and opened a seamless workflow between the underwriting team and all the data suppliers.
The Process:
- Each data supplier was given explicit instructions regarding the format, type, and precision of the data required.
- Suppliers could easily understand and meet these requirements using their existing tools and processes.
- Easy collaboration and cooperation in the data sourcing as gaps or missing data were quickly identified and either rectified or the data model was easily adjusted.
- All adjustments and agreements were documented within Verodat to maintain the context and integrity of the final data, which is vital for supporting AI implementation.
- This process instilled an ‘AI ready’ by design mentality. Verodat ensured the data flow adhered to the defined data context, a critical element in AI interpretation of data.
“ This has been such a simple and clear process from start to finish, using GPT to answer questions about my business is mind blowing, I can’t believe we achieved this in a matter of weeks.”
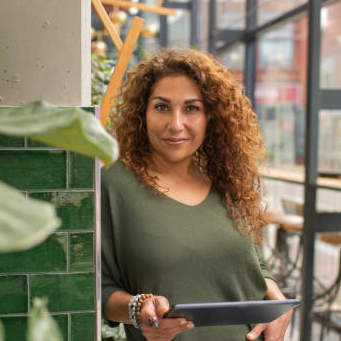
Automated and Accurate
The underwriting team relied on a steady flow of data from a multitude of suppliers, this was the cause of a critical roadblock in many of their previous data management projects. Verodat allowed the team to easily define who they needed the data from, what data they needed and how often they needed it. This allowed them to completely eliminate the manual back and forth of chasing data suppliers, the task now automated and managed securely within the platform.
The most crucial element of this stage however, was a clearly defined data structure, meaning that almost overnight, the underwriting team eliminated almost 90% of their time spent consolidating bordereaux files.
Ultimately, this automation and defined supply cadence created a data supply maintained with full information on the supply status- another vital element in the enablement of downstream AI. For example:
- Automated connector to Sharepoint folders
- Automated connection to binder management system
- Automated broker submission requests
- Broker submission portal for excel submissions
Another outcome of this stage has been vastly improved relationships between the team, brokers andother data suppliers, with the data supply chain offering a more seamless collaboration with clear expectations and schedules. It also allowed the business teams to take more control of the process without the high dependency on the technical teams.
Trusted, Traceable, Transparent.
As data flowed through the data supply chain, the underwriting team could easily identify, and instantly remedy, missing data, anomalies and errors. With data that was filtered, mapped, validated and transformed and any error handling along the process logged and tracked, enabling AI became a simple and fast project.
Used to conducting much of their reporting in Microsoft Excel, the underwriting team instantly switched on the Verodat Excel add-on which provided underwriters with access to clean, consolidated and reliable data in a familiar environment where they could independently perform data analysis.
A previous attempt to roll out Excel Co-Pilot within the team had stalled, due to the time-consuming manual activities required to consolidate data from different broker formats. The quality of the data was also causing significant challenges with underwriters unable to correct some issues without assistance from brokers. This resulted in too many errors to fully trust the outcome. With the new data layer in place, Excel Co-Pilot was also instantly switched on allowing them to quickly and easily apply complex formulas, build chart visualisations, and identify trends in their data.
When combined, the Verodat Excel add-on and Excel Co-Pilot enabled non-technical users in the underwriting team to build sophisticated dashboard visualisations for data analysis, and automatically refresh them with the latest data from brokers. In one click, the Excel add-on brings in the latest rows of data received since last refresh, automatically updating any associated pivot tables, charts and graphs in their dashboard reporting view.
This enabled users to independently deliver dashboard reporting solutions without depending on a data analytics team to build reports and react to changes.
AI You Can Trust.
Reiterating the goal of reaching a level of business understanding that allows them to rapidly react to both internal and external factors, further embracing AI was the next logical step for the procurement team.
Underwriting teams used a variety of easy to implement GPTs to perform analysis of underwriting data at a micro and macro level. This allowed the team to chat to their data in natural language and could instantly ask questions that would previously have required extensive analytics building, such as:
- Easy analysis of business lines and renewal and retention rates.
- Reviewing claim stats and trends.
- Loss ratios.
- Assess risk levels from external forces such as weather or market changes.
- Automate manual processes such as proposal processing.
- Suggesting recommended decisions based on previous accepted proposals.
- Analyse risk exposure across existing book of business and highlighting ways to reduce risk.
- Fraud detection and anomalies
Going one step further, with the right data now in place, a fine-tuned model (FTM) can significantly enhance an underwriting team’s capabilities by providing more specialised and context-aware assistance.
It can streamline the process further through precise automation of routine tasks like data entry, document processing, and prescriptive risk assessment, tailored to the specific needs and terminologies of the team.
Additionally, an FTM can offer deeper insights and more accurate predictions in areas like scenario and risk analysis.